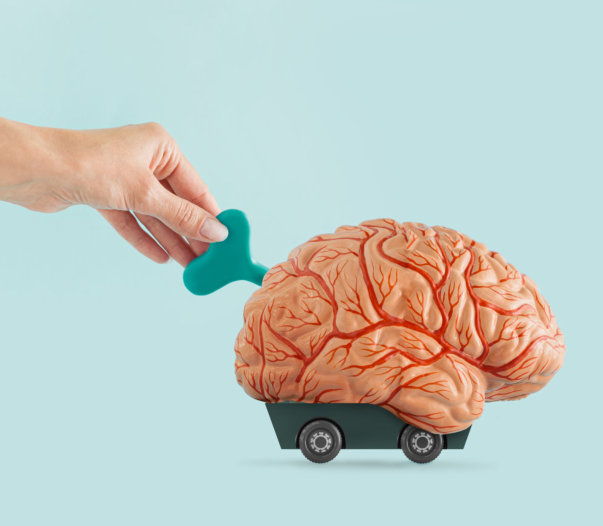
What makes you, well, you? Do you have a favourite TV programme? A specific style of dress? Do you purchase and consume all organic food? Furthermore, what do you like to read? How do you find enjoyment in your life? A number of data-churning services likely know these things about you, and they use this information to guess (fairly accurately) what you might want to do next.
Day-to-day patterns of behaviour and our social interconnectedness are all definable. Predictive algorithms are very skilled at forecasting our future actions and guessing what we might do next. This expertise makes them brilliant marketers, providing you access to something just as you desire it.
There is a long-standing idea of giving people what they want in a timely fashion. When I think about predictive algorithms in marketing, I can’t help but cast my thoughts back to a little invention called the ‘Teasmade‘—something I, as an expat in the UK, was introduced to when it was well past its peak marketability. This quirky and clever little device could make a cup of tea for you at the exact time you specified. Set the clock for 7 a.m., and just as you rise, a perfect brew is ready for you. It’s one of the early forms of robotic household gadgetry, and many are now likely gathering dust in cupboards throughout the UK.
Product designers and marketers have built upon this idea of timely and effective delivery as a way to generate sales for decades, if not since the inception of commerce. The difference now is that insight into the cross-section of desire and a feeling of need is powered by neural networks and AI. Sometimes our wants and needs appear as if by magic on the screen in front of us, and this is a strong driver of engagement.
The key difference between the power of the Teasmade to please its customers and AI and neural-network-backed marketing is the lack of consumer input. Thoughts and feelings are now understood so well by these behemoths that we no longer have to express what we want to be presented with our innermost desires.
But what do these systems really know about us, and how clever are they?
I believe there is a conundrum here. Data and analytics are powerful tools in prediction, but perhaps not so powerful in getting to the core of human psychology or, to expect more of them, improving the quality of life for the general population. It can be easy to latch onto the idea that now we have all this predictive data, we UNDERSTAND people and their motivators. But it’s here that we need to pause and think.
If your Teasmade made you a cup of tea at the right time every morning, would you drink it? Even if you didn’t really fancy it? Or fancy tea at all? Perhaps you would have really preferred coffee this morning, but would the act of presenting you with something you thought you wanted compel you to accept its solution, even if it didn’t entirely fit the bill?
To use a digitally informed example, if a medical app designed to aid diagnostics read your data, performed some analysis, and provided you with a diagnosis, would you accept it? And what about its proposed treatment plan? What if the diagnosis didn’t seem to totally fit your experience, but it felt close enough? What if the proposed treatment path felt limiting and narrow – but went just some way to address your concerns or issues? How far would you trust this predictive analysis? Could you trust the data this diagnosis was backed on to truly represent you – the individual? How much of the advice and treatment from this app would you accept? Would you feel equipped if you wanted to challenge that diagnosis and treatment?
Please don’t misunderstand me—AI in medicine is a game-changer for patient wellbeing, and I will cover that in future articles. When used intelligently, it can mean the difference between life and death for patients, or between living in pain and not. And it is exactly this type of empowerment that we should encourage technologists to focus on.
Another, perhaps less ‘life or death’ example, is music streaming apps. For the years I lived in London, it was necessary to download a playlist to listen to music when commuting on the Tube, due to the lack of reception. In all my laziness, I never downloaded more than one or two, usually switching to an audiobook if I grew bored with the same few songs.
When my reception returned, because of this Tube-travel-time playlist history, major music streamers continued to narrow the scope of new listening suggestions and, over time, whittled things down to such a narrow predictive selection that I felt like I was listening to the same song, on-repeat. The predictive analytics didn’t really understand me or what I actually wanted because my behaviour did not match my true interests or desires. But in that interim period, before I grew so frustrated that I gave up, I accepted a lot of ‘good enough’ song choices. They just weren’t exciting or truly what I wanted – even if I couldn’t precisely define what that was myself. I took what was on offer, but it didn’t challenge me or engage me in any meaningful way. It wasn’t able to ‘get’ me – the algorithms got in the way and left me in a ‘bland and acceptable’ place.
And I think there is something that is, at best, disquieting in this type of acceptance. That so passively I could have allowed technology driven by predictive analytics to make so many choices on my behalf like this. It doesn’t sit well in my psyche.
The complexities and quirks that make me ‘me’ and you ‘you’ are deeply intricate. There is a complex intersection between ‘need’ and ‘want’ at the heart of all of us. Just because the prediction of behaviour and outcomes is technically now well within our grasp, it doesn’t mean truly we understand ourselves – or each other. Yet. If we confuse accurate prediction with getting it ‘right’ for the customer, we might ultimately be doing more harm than good.